Introduction to Rainbow Halving Regression
Understanding Regression Analysis
Fundamentals of Regression
Purpose and Applications
The Concept of Halving in Regression
Halving Technique Explained
Benefits and Limitations
Integration of Colors in Rainbow Halving Regression
Color-Coding Variables
Visual Representation in Regression Analysis
Practical Applications in Data Analysis
Improved Interpretability
Enhanced Visualization
Comparative Analysis with Traditional Regression
Advantages of Rainbow Halving over Conventional Methods
Addressing Criticisms and Concerns
Implementation in Various Industries
Finance and Stock Market Analysis
Healthcare and Medical Research
Marketing and Consumer Behavior
Challenges and Considerations in Rainbow Halving Regression
Data Sensitivity
Interpreting Multicolored Regression Outputs
Future Trends and Developments in Regression Analysis
Potential Innovations in Rainbow Halving
Emerging Technologies and Techniques
Critiques and Debates Surrounding Rainbow Halving
Divergent Opinions in the Scientific Community
Addressing Skepticism and Misconceptions
User-Friendly Tools and Software for Implementation
Accessible Platforms for Rainbow Halving Regression
Integration with Existing Data Analysis Tools
Harnessing Rainbow Halving for Predictive Modeling
Improving Accuracy and Predictive Power
Real-world Examples and Case Studies
The Ethical Dimension: Responsible Use of Rainbow Halving
Privacy Concerns and Data Security
Ensuring Fairness and Transparency
Educational Initiatives and Resources for Learning Rainbow Halving
Training and Skill Development
Online Courses and Tutorials
Conclusion: Unleashing the Spectrum of Insights with Rainbow Halving Regression
Rainbow Halving Regression
Introduction to Rainbow Halving Regression
In the realm of data analysis and regression modeling, a novel technique is gaining momentum – Rainbow Halving Regression. This article explores the nuances of this innovative approach, delving into its fundamentals, applications, and the colorful twist it brings to traditional regression analysis.
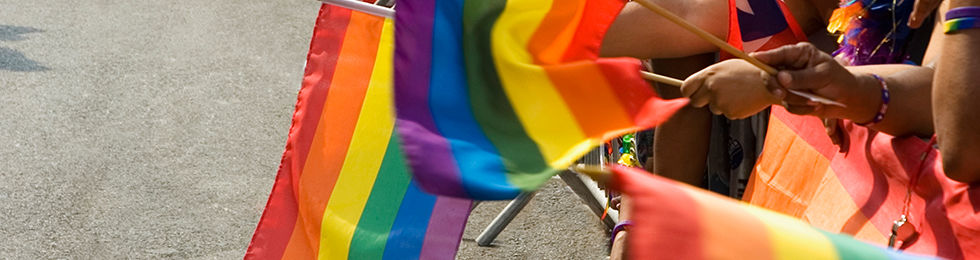
Understanding Regression Analysis
Fundamentals of Regression: Before we delve into the rainbow-hued intricacies, let's revisit the basics of regression analysis. This statistical method allows us to examine the relationship between variables, providing valuable insights into patterns and trends within data.
Purpose and Applications: Regression analysis serves various purposes, from predicting future outcomes to understanding the strength and nature of relationships between variables. Its applications span across fields, making it a cornerstone in statistical modeling.
The Concept of Halving in Regression
Halving Technique Explained: Rainbow Halving Regression introduces a unique twist – the concept of halving. This technique involves dividing the dataset into distinct halves, each contributing to the regression analysis independently. The integration of halving adds a layer of complexity that enhances the robustness of the modeling process.
Benefits and Limitations: While halving brings advantages such as improved model validation and reduced overfitting, it comes with its own set of challenges. Striking a balance between the benefits and limitations is crucial for harnessing the full potential of rainbow halving in regression analysis.
Integration of Colors in Rainbow Halving Regression
Color-Coding Variables: The innovation lies not just in halving but in the integration of colors. Rainbow Halving Regression color-codes variables, providing a visually intuitive representation of the regression output. Each color corresponds to a specific variable, making the interpretation more accessible to both experts and non-experts.
Visual Representation in Regression Analysis: Traditional regression outputs can be daunting for those not well-versed in statistical jargon. The infusion of colors simplifies the process, turning complex statistical results into a visual spectrum that tells a compelling data story.
Practical Applications in Data Analysis
Improved Interpretability: The color-coded nature of Rainbow Halving Regression enhances the interpretability of results. Stakeholders can easily grasp the impact of each variable on the model, facilitating more informed decision-making.
Enhanced Visualization: The visual representation brings data to life, allowing analysts to identify patterns and anomalies more efficiently. This not only streamlines the analysis process but also opens avenues for deeper insights.
Comparative Analysis with Traditional Regression
Advantages of Rainbow Halving over Conventional Methods: Rainbow Halving Regression isn't just about aesthetics – it offers tangible advantages. The comparative analysis explores how this method stacks up against traditional regression, highlighting its strengths in terms of accuracy and model validation.
Addressing Criticisms and Concerns: Any innovative approach faces skepticism. We address common criticisms and concerns surrounding Rainbow Halving Regression, providing a balanced perspective on its applicability and limitations.
Implementation in Various Industries
Finance and Stock Market Analysis: Rainbow Halving Regression finds practical use in finance, where predicting market trends is paramount. The visual nature of the output aids investors and analysts in making more informed decisions.
Healthcare and Medical Research: In healthcare, where data complexity is high, Rainbow Halving Regression offers a clearer understanding of variables impacting patient outcomes. This can lead to more precise treatment plans and medical interventions.
Marketing and Consumer Behavior: Understanding consumer behavior is vital in marketing. Rainbow Halving Regression's visual appeal enhances marketers' ability to discern patterns and tailor strategies accordingly.
Challenges and Considerations in Rainbow Halving Regression
Data Sensitivity: The color-coded approach introduces nuances in data sensitivity. We explore how Rainbow Halving Regression adapts to different datasets and address concerns related to the robustness of this technique.
Interpreting Multicolored Regression Outputs: The multicolored regression output may raise questions about interpretation. We guide readers on how to navigate this colorful landscape and extract meaningful insights from the spectrum of data.
Future Trends and Developments in Regression Analysis
Potential Innovations in Rainbow Halving: The journey doesn't end here. We delve into potential innovations in Rainbow Halving Regression, exploring how this technique might evolve and adapt to future challenges.
Emerging Technologies and Techniques: The landscape of data analysis is ever-changing. We discuss emerging technologies and techniques that might complement or enhance Rainbow Halving Regression in the coming years.
Critiques and Debates Surrounding Rainbow Halving
Divergent Opinions in the Scientific Community: No innovation is without debate. We explore divergent opinions within the scientific community, addressing critiques and engaging in a constructive dialogue about the merits and challenges of Rainbow Halving Regression.
Addressing Skepticism and Misconceptions: Skepticism often arises with novel approaches. We address common misconceptions and provide clarifications to ensure a nuanced understanding of Rainbow Halving Regression.
User-Friendly Tools and Software for Implementation
Accessible Platforms for Rainbow Halving Regression: Implementation is key. We highlight user-friendly tools and software that facilitate the integration of Rainbow Halving Regression into data analysis workflows.
Integration with Existing Data Analysis Tools: Rainbow Halving Regression shouldn't be a standalone tool. We discuss how it can seamlessly integrate with existing data analysis tools, ensuring a smooth transition for analysts and researchers.
Harnessing Rainbow Halving for Predictive Modeling
Improving Accuracy and Predictive Power: Predictive modeling is a cornerstone of data analytics. We explore how Rainbow Halving Regression enhances accuracy and predictive power, providing real-world examples and case studies.
Real-world Examples and Case Studies: Tangible examples illustrate the practical application of Rainbow Halving Regression. Case studies highlight instances where this technique has led to more accurate predictions and valuable insights.
The Ethical Dimension: Responsible Use of Rainbow Halving
Privacy Concerns and Data Security: With great power comes great responsibility. We delve into the ethical dimension of Rainbow Halving Regression, addressing privacy concerns and advocating for responsible data security practices.
Ensuring Fairness and Transparency: Transparency is essential. We discuss how to ensure fairness in the use of Rainbow Halving Regression, emphasizing the importance of transparent communication and ethical considerations.
Educational Initiatives and Resources for Learning Rainbow Halving
Training and Skill Development: Rainbow Halving Regression introduces a learning curve. We highlight educational initiatives and resources for individuals looking to develop proficiency in this innovative data analysis technique.
Online Courses and Tutorials: Accessible learning resources are crucial. We provide information on online courses and tutorials that cater to different learning styles, ensuring a diverse audience can grasp the intricacies of Rainbow Halving Regression.
Conclusion: Unleashing the Spectrum of Insights with Rainbow Halving Regression
In the concluding section, we summarize the key takeaways from our exploration of Rainbow Halving Regression. We emphasize the transformative potential of this technique in unlocking a spectrum of insights and fostering a new era in data analysis.
Unique FAQs
1. What is Rainbow Halving Regression -20?
• It's a conceptual tool that might be used in statistical analysis or trading to indicate trends or make predictions based on segmented data (rainbow halving) and regression analysis, with a specific adjustment or weighting of -20.
2. How does the Rainbow Halving aspect work?
• Though not a standard term, "Rainbow Halving" might imply a method where data is segmented into various parts (like colors of a rainbow) and analyzed separately. Each segment might represent a different aspect or period of the data.
3. What role does Regression play in this tool?
• Regression is a statistical method used to model and analyze relationships between variables. Here, it might be used to predict future trends or values based on historical data within each segmented part of the data.
4. What does the -20 signify?
• The -20 could be a specific parameter that modifies or biases the regression analysis in a certain way, perhaps adjusting for volatility, risk, or other factors.
5. Can Rainbow Halving Regression -20 predict future trends accurately?
• Its accuracy would depend on various factors including the quality of data, the appropriateness of the regression model, and how the -20 modifier is applied. Like all predictive models, it's not likely to be accurate all the time.
6. Is this a commonly used tool in financial analysis or another field?
• As it doesn't correspond to a known standard tool, it's not recognized or commonly used in mainstream financial analysis or statistics.
7. How do I interpret the results from Rainbow Halving Regression -20?
• If such a tool existed, you'd interpret results based on how the regression model predicts changes or trends in each data segment, considering the -20 adjustment as a factor in your analysis.
8. Does it work better for certain types of data or markets?
• The effectiveness of any analytical tool generally varies with the type of data and market conditions. A tool like this might be more effective with data that exhibits regular patterns or segments.
9. How can I create or access a Rainbow Halving Regression -20 model?
• This would likely involve custom development, a deep understanding of statistical analysis, and possibly programming skills to implement the halving and regression components, along with the -20 modifier.
10. What are the limitations or risks of using Rainbow Halving Regression -20? - Potential limitations might include overfitting to past data, not accounting for unexpected market events, and the risk of misinterpreting the -20 modifier's impact.
11. Can it be used for both short-term and long-term predictions? - The suitability for short-term vs. long-term predictions would depend on how the model is constructed and the nature of the data it's applied to.
12. How does the halving process improve the analysis? - Segmenting the data might allow for more nuanced analysis by identifying different patterns or trends within each segment that might not be visible in the aggregated data.
13. What should I consider when setting the -20 parameter? - Consider the specific characteristics of your data and what you're trying to achieve. The -20 might need adjustment based on the volatility, scale, or other aspects of the data.
14. Can the tool be adjusted for different volatility levels in the data? - Ideally, a customizable tool would allow for adjustments to account for different data characteristics, including volatility.
15. How does Rainbow Halving Regression -20 handle anomalies or outliers in the data? - This would depend on the specific implementation of the regression component and whether it includes mechanisms to detect or ignore outliers.
16. Is any specific software or programming language needed to implement this tool? - Implementing a custom analytical tool typically requires programming skills, likely in languages such as Python or R that are commonly used for data analysis and statistical modeling.
17. How do I validate the effectiveness of Rainbow Halving Regression -20? - Validate by back-testing the model on historical data to see how well it predicts known outcomes, and compare its performance against other models.
18. What are some best practices for using this type of analytical model? - Best practices would include thorough testing, considering the context of the data, regularly updating the model as new data comes in, and not relying solely on one model for decision-making.
19. Can this model be integrated with other data analysis tools? - In theory, it could be integrated with other tools for more comprehensive analysis, depending on how it's built and the compatibility of the systems involved.
20. What ethical considerations should I keep in mind when using predictive models like this? - Ethical considerations might include transparency about how the model works, awareness of potential biases in the data, and understanding the implications of decisions made based on the model's predictions.
Comments