Introduction:
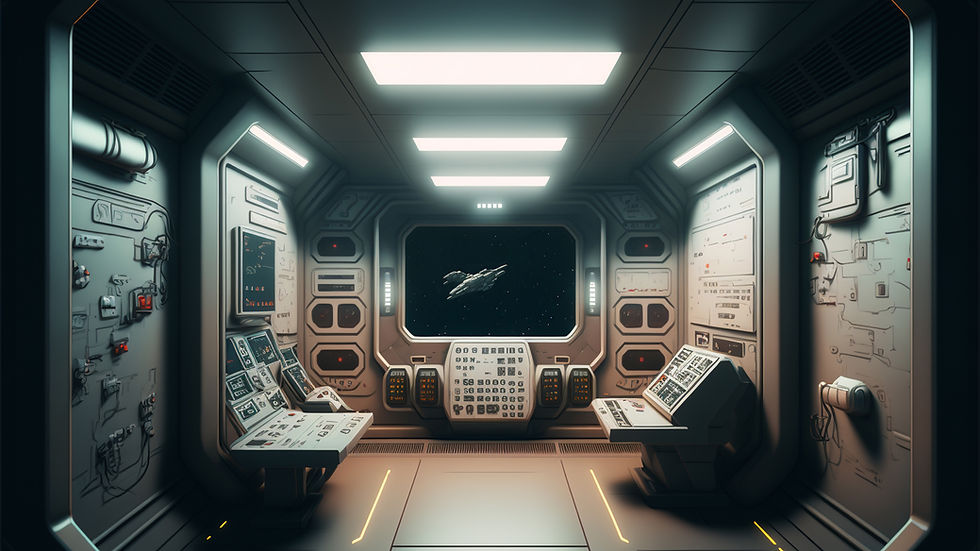
(AI) and Machine Learning (ML) have emerged as transformative forces in the realm of support processes, particularly in Level 2 (L2) and Level 3 (L3) support. This examination explores how AI and ML are automating tasks, augmenting decision-making, and optimizing support services for enhanced efficiency.
Automation in L2 Support Processes:
1. Automated Ticket Routing:
Role:
AI algorithms can automatically route support tickets to the most appropriate L2 support agent based on the ticket's content, urgency, and the agent's expertise. This minimizes response times and ensures tickets are handled by the most qualified personnel.
2. Chatbot Assistance:
Role:
AI-powered chatbots handle routine queries and frequently asked questions in real-time. This not only provides immediate assistance to users but also frees up L2 support agents to focus on more complex issues.
3. Predictive Issue Resolution:
Role:
ML algorithms analyze historical support data to predict potential issues and recommend solutions. L2 support can proactively address emerging problems, reducing the number of support tickets and improving overall user satisfaction.
4. Automated Documentation Updates:
Role:
AI tools can automatically update documentation based on resolved support cases. This ensures that knowledge bases are always current, aiding both L2 agents and users in finding accurate and up-to-date information.
Enhancements in L3 Support Processes:
1. Root Cause Analysis:
Role:
ML algorithms perform root cause analysis on complex issues, identifying underlying problems more quickly and accurately than traditional methods. This accelerates the troubleshooting process in L3 support.
2. Dynamic Ticket Escalation:
Role:
AI can dynamically assess the severity of a support ticket and automatically escalate it to L3 support if needed. This ensures that critical issues are addressed promptly by the most specialized team.
3. Performance Analytics:
Role:
ML-driven analytics provide insights into system performance, helping L3 support identify potential bottlenecks, vulnerabilities, or areas for improvement. This proactive approach enhances system reliability and stability.
4. Continuous Learning Models:
Role:
ML models learn from resolved L3 support cases, adapting and improving over time. This continuous learning enables the system to handle evolving technologies and challenges, contributing to more effective long-term support.
Overall Impact on Support Processes:
1. Faster Problem Resolution:
Impact:
Automation and predictive capabilities significantly reduce the time taken to identify and resolve issues in both L2 and L3 support. Users experience faster problem resolution, leading to increased satisfaction.
2. Resource Optimization:
Impact:
Automation allows support teams to allocate resources more efficiently. Routine tasks are handled by AI, allowing human support agents to focus on high-value, intricate issues that require human expertise.
3. Improved User Experience:
Impact:
The combination of AI and ML enhances the overall user experience by providing quicker responses, personalized support, and proactive issue resolution. Users feel more supported and valued.
4. Data-Driven Decision-Making:
Impact:
AI and ML empower support teams to make informed decisions based on data analysis. This data-driven approach ensures that support processes are continually refined for optimal performance.
Conclusion:
Artificial Intelligence and Machine Learning have become indispensable tools in automating and improving L2 and L3 support processes. The impact is profound, leading to faster problem resolution, resource optimization, enhanced user experiences, and a data-driven approach that ensures ongoing improvements in support services.
Comentarios